AI series part two: How artificial intelligence and machine learning fit into your fuel pricing strategy
As your market progresses through phases of maturity, you must measure the value of your machine intelligence carefully. More specifically, there will be varied and potentially extreme changes occurring in the market during the transition from stage two of maturity (unstable) into stage three (competitive). As you set prices, your competitors will react; as the number of new entrants into the market rises, your supply costs may change. You should begin to consider how to incorporate machine learning and artificial intelligence into your strategy as these changes occur. Good news: You don’t have to worry. You have to learn.
The proliferation of data we explored in the last post will allow you to react appropriately to the shape of the morphing market. There will, of course, be intake, learning, and action. The optimization aspect of your pricing strategy will be based on this machine learning.
Step by step, this occurs as follows:
You (via an automated process) will feed data (your own prices, competitor prices, your volumes, your costs) into your system, at the required frequency. The system will use this data to refine the model, responding to demand shifts — the right machine learning model will understand how demand has varied as your prices, competitors and market have. Each time new data is fed into the system, it is asking the questions: did we perform as anticipated based on that price? Does the model need to be refined based on this performance? This model refinement is a continual process of learning and adjusting.
Using this model, and with consideration for your price positioning strategy, volume and margin targets and price-move constraints, the optimization algorithms will be able to say, “Based on what I know about everything I see, I know what the optimal price should be.” This is where artificial intelligence comes into play: when retailers set their prices while considering multiple constraints or rules that would have been taken into account if they were manually making that pricing decision. For example, among other things, you will need to think about what the site down the road is doing in order to stay within a given range. You will need to consider how a cost may change, and how that change will affect your margin. You will need to consider the perfect price that allows for you to gain volume without gaining so much that you run out of fuel. You will need to consider how to avoid eroding the market or initiating a price war. All while ensuring that you are meeting your margin and volume targets. Artificial intelligence is the act of performing checks based on these rules to produce a price that is optimal.
All of this can feel decidedly objective. As we mentioned in the introduction to this piece, however, caution is a requisite. There will always remain subjectivity if you are to reach pricing success — without contributing too greatly to volatility. You must, therefore, create exceptions. There will be instances where all checks have been run, an optimal price has been produced, but a particular case requires handling as an exception. At that point, it’s up to you to make the subjective decision that informs the model.
Generally, we see at least an 80-90 percent automation rate. But there may be around 10 to 20 percent that you want to view a bit more deeply — perhaps because this particular site is your “pet” site, or perhaps because you know this site has been low performing and you want to keep your eye on it. Or perhaps there are secondary rules that shouldn’t constrain the optimization process, but you want to be informed if they are not being met. That’s where exceptions come into play, and where you should exercise your informed subjectivity.
In our next post, we’ll discuss the intricacies of maintaining your pricing strategy while simultaneously navigating your market maturity stage — all using artificial intelligence and machine learning.
Read more articles about:
UncategorizedSubscribe and get the latest updates
You may unsubscribe from our mailing list at any time. To understand how and why we process your data, please see our Privacy & Cookies Policy
Related blogs
Uncategorized
AI series part one: How artificial intelligence and machine learning have advanced with data proliferation
In our three-part series on artificial intelligence and machine learning, we'll help you understand the differences...
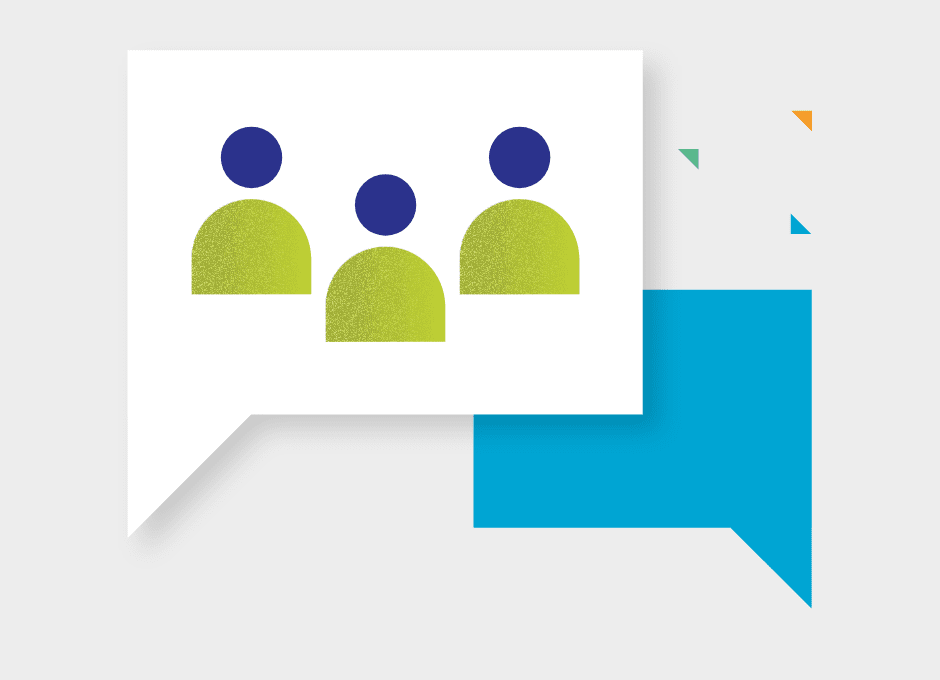
Uncategorized
AI series part 3: How much should you rely on artificial intelligence in fuel pricing?
As we mentioned in part two of this series, blindly relying on computational processes and models for pricing,...
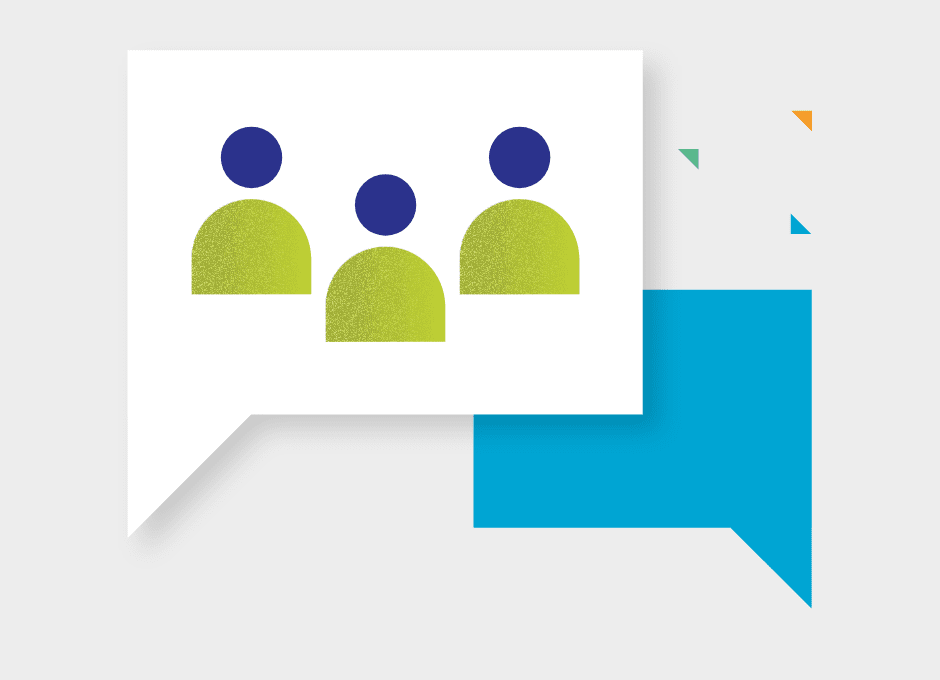